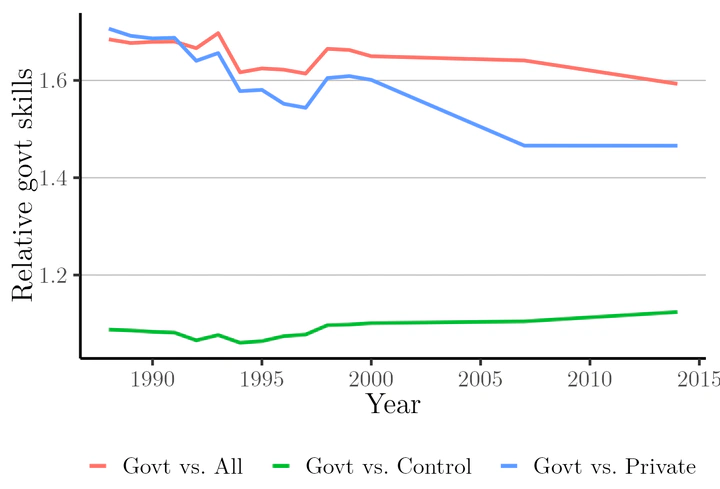
This paper provides a new approach to estimate government worker skills that is applicable in settings where government output is unobserved and government wages are uninformative about skill differences. The approach estimates skills from wages in comparable jobs in the private sector, relates these skills to skill-related observables using Machine Learning tools and then predicts government worker skills out-of-sample. I apply the new estimation approach to rich Indonesian household-level panel data from 1988 to 2014, showing two main applications. First, I show that the government worker skill premium has declined continuously over time. While government workers are highly selected, their skills have increased less strongly than skills in the overall population, driven by the most skilled workers ending up in the private sector. Second, I analyze government wage setting: the Indonesian government pays a wage premium of at least 30% conditional on skills, about 1/3 of which is driven by the large gender wage gap in Indonesia’s private sector.